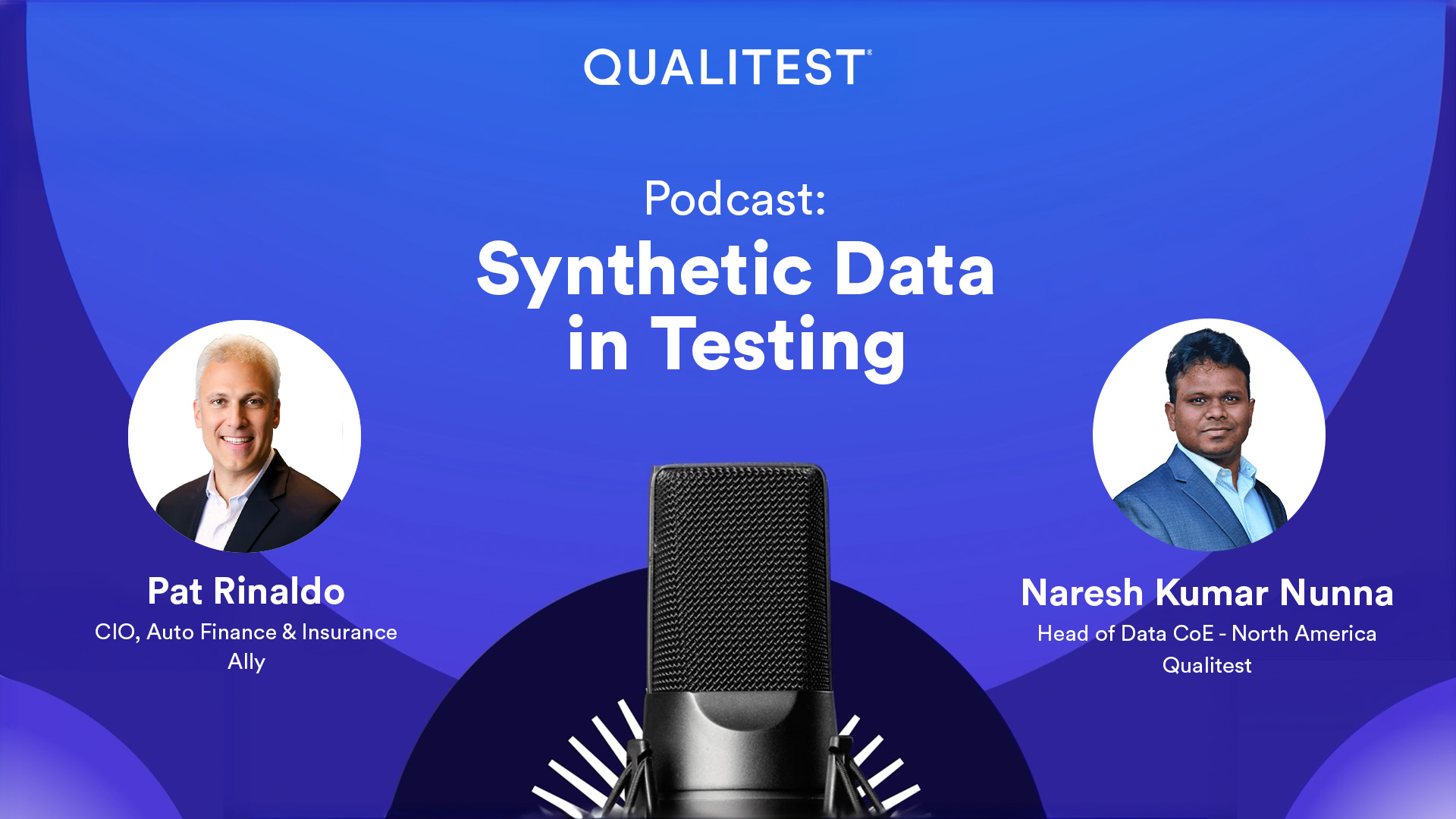
Panelists
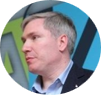
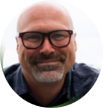

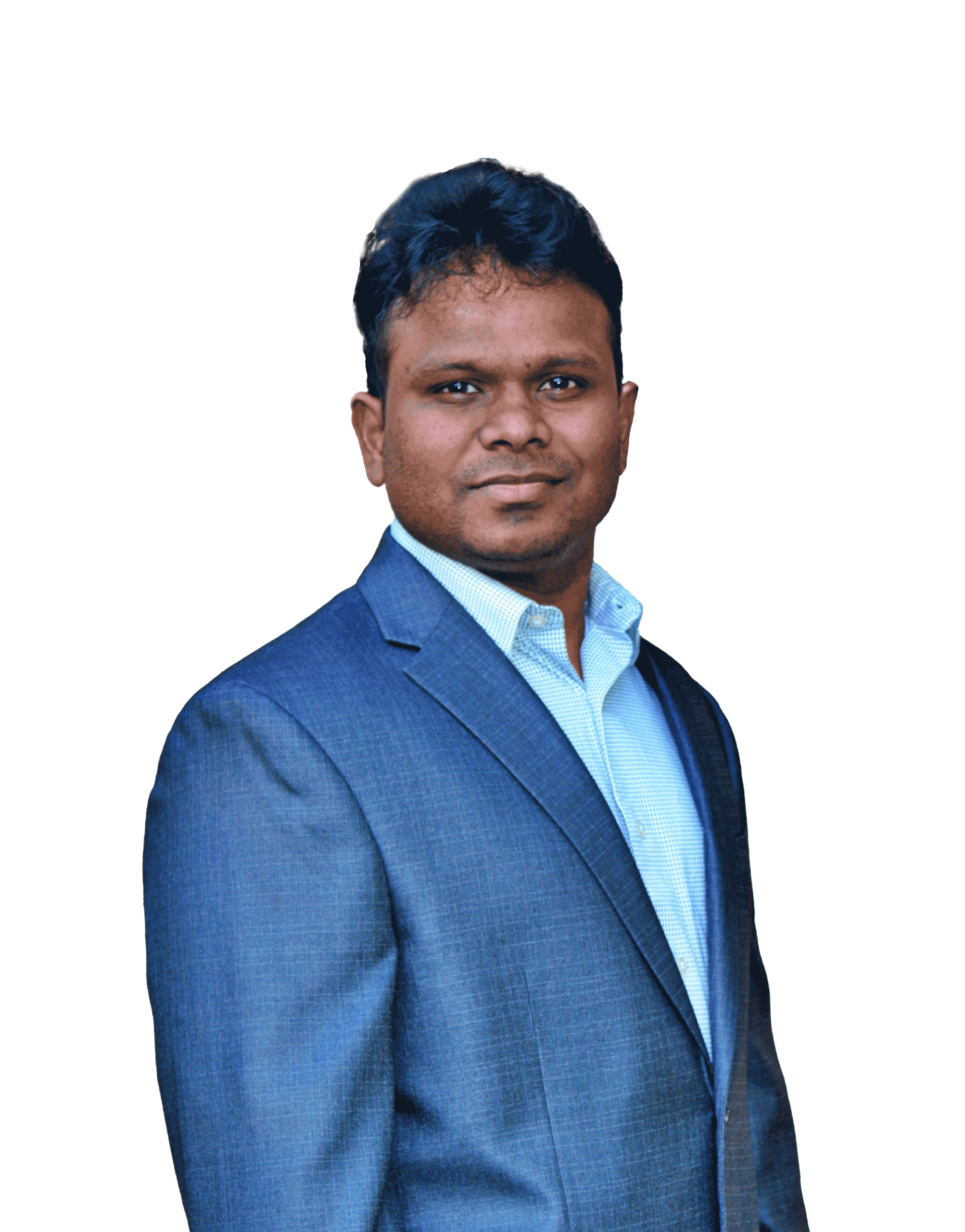
Michael Larsen (INTRO):
Hello and welcome to The Testing Show.
Synthetic Data in Testing.
This show was recorded on Tuesday, April 15th, 2025.
And with that, let’s get on with the show.
Matthew Heusser:
Well, hello and welcome back. This time on The Testing Show, we want to take a different topic that’s coming increasingly important, which is synthetic test data. If you work in any kind of large customer data environment, increasingly you can’t use the real data because of problems with privacy. But if you fake up your own data and it’s limited, it’s not a representational sample, how do you do both? Qualitest has had some success working with partners through that process and we’ve got a real case study to talk about today with real experience and to talk about that today we’ve got a real use case with a working customer and that is Pat Rinaldo. He is the Chief Information Officer for the automotive and insurance divisions of Ally Bank, located close to me, Detroit, Michigan and welcome to the show, Pat.
Pat Rinaldo:
Yeah, thanks. Glad to be here.
Matthew Heusser:
Did I get anything wrong in that?
Pat Rinaldo:
Well, you got it right now. My full name is Pasquale. I should have made you say that because you would’ve never gotten that right, but I gave you the short version, so no problem at all. You got it.
Matthew Heusser:
Thanks. And we also have… and Qualitest has been working with Ally Bank for some time and we have Naresh Kumar Nuna who’s an associate vice president with Qualitest focusing on synthetic data and cloud. Did I get that right?
Naresh Kumar Nunna:
Yes, you did. You did.
Matthew Heusser:
And you’re coming to us today from Dallas?
Naresh Kumar Nunna:
Yes, I’m in Dallas.
Matthew Heusser:
Okay. Is the team that’s working on this, do we have people in Detroit? We have people all over.
Naresh Kumar Nunna:
It’s mostly we are still embracing the remote culture, so we have people definitely working across North America, India, different locations
Matthew Heusser:
And as always we have Michael Larsen with us. Morning Michael. Good time zone.
Michael Larsen:
Good time zone is right. Good to be here. It is 6 0 9 where I am right now. So good morning and there’s no sunlight yet.
Matthew Heusser:
So did I miss anything about synthetic test data and why it’s important?
Pat Rinaldo:
I think you hit it well for us, that foundation and data protection and data security and ensuring the sanctity of our customer’s data was already in place has been for a long time. What we struggle with is the coverage, ensuring that we have enough test data to actually prove out what we need to prove out to be able to validate our systems, especially when you’re going into something that is brand new and you don’t have any sample data or any examples of how it might look. So that’s been a challenge for us. It’s a lot of effort to really develop through manual methods, the type of data that you might need to move quickly and so we see synthetic data as that enabler for how we’re going to be able to innovate at the rate that we need to innovate. Naresh, can you add anything to that?
Naresh Kumar Nunna:
Yeah, I think you summarized it well. I think I would say Ally is definitely way past the line, way past maturity in terms of their journey with the test data management. I think there is three things which I would probably look at what I’m observing with Ally and outside as well. Mostly if you’re focusing on automation, focusing on ai, focusing on operational efficiency, synthetic data is the way to go. It’s really not touching all the key essentials ingredients, which a lot of business are looking for today.
Matthew Heusser:
How did that go when Qualitest started working with Ally? How did we start this partnership together? Where did we come from to get where we are today? It wasn’t overnight, right?
Pat Rinaldo:
I take a long time to get comfortable with people and with some of the groups that we work with. We started working with Qualitest a couple of years ago. They brought a lot to the table in terms of thought leadership in the testing space. Originally we started in smaller more consulting type agreements and started to expand into spots where they were taking more ownership of individual pieces of testing. And through that journey, that really helped us to understand how their culture fit with ours, how their ambition and ways of working fit with ours. And we accelerated the relationship in at the end of 24 where they took on a managed testing service for one of our most significant application spaces, and that really gave them a strong foothold and really helped them help us to accelerate our journey, accelerate the quality of our testing. And a big part of that has been the mentoring and thought leadership that they’ve provided as it relates to synthetic test data. We had already started down that path, especially in our analytics and reporting area where we’re able to, through our own models, build the type of synthetic data that we need, but we hadn’t really stepped into the application space and being able to synthesize full auto loans and the types of data that we need, and so that’s where they’ve been able to lean in and help us quite a bit.
Michael Larsen:
So if I could step in here again, I oftentimes take on the role of the beginner of a given area and this fascinates me. So for those people who may not really understand, test data, is test data. Why are we making a distinction here between test data and synthetic test data? In other words, what is the distinction, and again, I want to present this from a perspective of some people might not understand the distinction and why it’s important.
Naresh Kumar Nunna:
Yeah, absolutely. I can take that. Test data traditionally has been sourced from production environments, production staging environments. Generally you copy it, you mask it, and you provide a copy of deed by data in the lower environments, whether it is dev of QA performance or capacity environments. I think with synthetic data it’s a bit different in terms of we use algorithms, we use statistical models, we use machine learning techniques to create lookalike data, which is artificially generated, but it is very close resemblance to what our real data looks like from real world events, real world characteristics, real world property standpoint, what I would call it as a digital twin, which is made out of systematic algorithms.
Matthew Heusser:
So that sounds like a lot to me. Using machine learning to analyze the real data, to come up with predictive algorithms to create the fake data. So one of the things that frustrates me, there’s a book called “The Phoenix Project”, and if you read it very carefully, it’s everything is terrible, everything is terrible, everything is terrible. The hero comes in six months later, everything’s fine. That book should have been about those six months. Speaking of which, what were the barriers you found when you tried to do this and how did you overcome them?
Pat Rinaldo:
Like most technology projects or any type of initiative, you have the human barriers and you have the technical barriers. On the human side, people are comfortable with a certain way of working. They’re comfortable with the risk they’re taking or how they’re accomplishing their day-to-day work, and they’re often skeptical of something new. For us, we’re an agile shop, so we’re constantly learning. We’re constantly innovating and improving that mindset to take on new technology. Not only is it part of the DNA of my team, but it’s part of the DNA of all. We’re constantly innovating and we have a long track record of that, starting with that base of innovation, that base of continuous improvement that helped us on the human side, but you still have to tackle the change management. You still have to prove to people that you can be successful and you have to be able to explain to them what it means to them.
On the technical side, when you think about the duration of an auto lease or the duration of an auto loan or an insurance policy, those are many, many years. You’ve got in the US 50 different states to deal with different regulations everywhere. So when you look at a loan that might be in its first six months or it’s two years in or it’s five years in and the person went through bankruptcy or what have you, there’s a tremendous variety in that data and being able to synthesize that and create something that truly is realistic and that the systems believe is something that grew up within them and developed within them in the same way a customer would. That is extremely challenging, and so being able to get all of the data that we needed to profile, being able to start building those models, we’re still in the midst of it, but it is definitely an uphill climb, but the payoff is fantastic. I don’t know, Naresh, I probably simplified it way too much.
Naresh Kumar Nunna:
I think you covered, I was actually thinking about like five folds. One is the quality aspect of it. I have the data but do have enough of it from a coverage perspective. I want to test not just a positive scenarios. I also want to test the boundary scenarios. Edge case scenarios, or things I might not find in data, which copies that I use today, which is obviously limiting my testing and there is possibility I could find these events only in production, which is not what our businesses, our customers are looking for. I think speed aspect of it, I think you covered it as well. We are no longer talking about monthly and quarterly releases. We are talking about intra-day releases, weekly releases, and if I go with the traditional methods, if it takes too long for me to procure the data, then when I’m going to test it, when I’m going to release it.
The team piece, which you also touched is the culture. We’ve been used to this traditional methods of using test data. Do I really need to change it? I have so many responsibilities. Me as a tester, I have to write the automation scripts. I have to understand the requirements. I have to execute test cases, I have to retest it. The defect management, I have so many things I have to do already why I have to do one more activity, which is again, another mindset shift. Thinking more of this as an enabler, it’s not an additional work. Yes, it might feel initially as an additional work, but you do this today if you are a tester. There’s been a lot of studies audit of 60% of the time tester spending today on preparing test data, creating and conditioning test data
Matthew Heusser:
For sure.
Naresh Kumar Nunna:
That’s where I feel it’s becoming a barrier, but I think where I’ve seen which Ally, they already had some of those things addressed and it’s just a matter of showcasing the people with small successes. The use case, which Pat elaborated explained very clearly. I don’t just want the data. I want the data as if it looks like I would been like six months, one year, two years. It’s been really a difficult journey, but I think we are getting there,
Michael Larsen:
So if I can interject here just a little bit, this is something that intrigues me because of a project that I’m currently working on and one of the interesting challenges that we face in the sense that right now, and I think I’m on safe ground to say this, I’m not sharing any secrets. I’m working on a pretty big internationalization project, which means that a lot of the data that we are currently working with is going to be directed to multiple locales, is going to be directed to multiple countries, multiple alphabets, multiple examples, and one of the challenges that we face, of course with this is the data that you tend to put in, especially if you’re pulling information from a database. If that data is structured a particular way or it’s structured for let’s say the US market, is it still going to be fit for use if you apply it, say in India or if you apply it in Japan or China, but I mean help me out here.
That is a reality that I face a lot of the time, when I’m doing and examining translations and I have to stop and pause and say, hold on, why is, oh, right, that’s coming from the database that’s being pulled in X, y, blah. Okay, but let’s say that you need to make sure that you have a seamless integration with multiple locales with your data. Now, this might be beyond what I’m talking about here, but I’m just looking at it as an expanded use case. How would you approach something like that? Is that something that’s in the scope of what you’re doing or am I talking about something totally different?
Naresh Kumar Nunna:
It’s within the scope of what you’re talking about as well, different use cases, but one thing you did talk about is when I have a data today, maybe a lot of data, what I have is based off of English as a primary language. I might not have data for certain customers at certain events. That might have happened at a different location or a different region, so that’s where synthetic data comes into picture. It doesn’t have to be the data that I already have because it’s all generated. There are ways to do it. This is how I’m going to look like from a structure standpoint. This is the language and this is the type of data I’m looking for. If you can define in a simple structure and plain English, you can generate it or if I already have what I’m looking for, maybe it’s in a different language.
Can you take this as a sample and do it? Yes, that’s doable as well, right, so that’s where the technology has evolved compared to what we were seeing. While syn data has been there for more than two decades, the technology, what we have seen in the last five years is significantly matured. It can do it with samples, it can do it with just plain vanilla structure and schema definition, which could be used for wide variety. One thing we use when we are training Google search, you need data. When somebody is searching in their own locals, you need data to train them and there is only limited searches would’ve happened in the history for their particular language, but I’m testing this new translation engine, where do I get the data from? If you look at even further, a lot of these autonomous vehicles that are coming out in the market, they don’t have enough history of data to test their vehicles. There’s a lot of data hypothetical scenarios that’s been generated using machine learning algorithms and systematic algorithms to create the data. The applicability has been experiential. We’re not only talking about traditional software testing. We are doing for product. We are doing for IoT devices, we are doing for autonomous vehicles and so many more.
Pat Rinaldo:
Michael is definitely working on some interesting things, so maybe a little beyond what I have to tackle, but we do have similar challenges in terms of talk about auto loans in the 50 different jurisdictions, so the attributes are different throughout all of these different areas, but the basic concept of you have a car and you’re making a payment, that’s pretty clear. We all understand that that’s the day-to-day. Being able to take that and extrapolate it into how that should be represented, what the component parts are across all of these geographies. It’s not that different than what you’re talking about and it is something that we’ve been able to solve.
Matthew Heusser:
Another piece to that, when it comes to testing with synthetic data, let’s say you succeed, we’ve modeled a portfolio of 1000 different loans. We think these loans realistically are similar to what our customers do. We’ve got maybe it’s 10,000. We’ve got all of our jurisdictions in there, we’ve got positive cases, we’ve got negative cases, we’ve got combinatorics, we’ve got it all. Then somehow we have to do whatever we do, the processing of the payment. This month some people are even later, some people are late, some people pay it on time, some people didn’t pay enough, some people paid too much, adjust their balances and see where they end up, and then we got to know if the adjusted balance is correct, so we need an oracle to say, did you get the right answer with your synthetic data when you ran it through your modified program with all the changes that you made in the past two weeks? To get there, I think we need to talk about what is the core concepts of synthetic data, maybe a little bit on why it’s becoming critical.
Naresh Kumar Nunna:
There’s a lot of definitions out there in terms of what is synthetic data, how it should be. The way I look at is how real it is compared to what you’ve been used to. Is it allowing me to test what I want to test and is it allowing me to test, which I did not do it before. What I’ve been used to it test before is all positive scenarios, things which I know, but there is a lot of unknown things. For example, if you take fraud scenarios or AI/ML scenarios that are very, very complex, if you keep testing the product using a transaction which has already occurred in production, that means something already happened. You’re only testing and detecting on history, but fraud is always about prediction. In future, there is millions ways. How can I make my programs my core, my products more futuristic?
That’s where you have to be a little more creative than what you’ve been used to. It really needs to represent the real world scenarios, not in the history what could possibly happen in future. That’s one of the key aspect which we see when we are creating synthetic data solution. Second, it’s privacy by design. What happening is today all the regulated customers, too much of focus to the privacy, sum compliance. There is so much of control, so much of governance. What if for all of those innovators, I will give you data which is privacy free. Just imagine how much less pressure they feel now they’ll just only focusing on innovation. I just need to solve this business problem. Less bother about the privacy and regulations which are tightening and growing every single day. The third is the control aspect. It’s not just one size fits all.
If I’m testing a machine learning model, I need one type of test, one type of data. If I’m testing an API, if I’m testing a cards product or if you go by the test phases, if I’m doing a unit testing, component testing, integration testing or UAT, all of those require different sets of data, different permutations of the data, and you need to have as a human, the power of what you need and how much you need so that way you will specifically test what you intended rather than trying to figure out, I already have this data, how can I make my testing work? The fourth element is while we are using this data, there is also, especially in the traditional software side, and we also use it in the machine learning model training and finding the testing and efficacy and efficiency of those models, there might be some bias as well.
Sometimes you wanted to create the bias to see how your model is performing. These are real world scenarios again, when I have these biases where I’m looking for some type of customers with certain age ranges, if you suddenly give somebody who is 250 years old, that’s outlier one as an example. Second, you don’t always want to give the data, which is all only with one gender, one ethnicity. When you build this product, these builds for multiple people irrespective of their origins, irrespective of ethnicities. You need to have the ability to test those boundaries of those machine learning models as well. Lastly, how quick I can do it, the on demand is what a lot of today’s DevOps is looking for. Create, test, validate, touch, and then redo. That’s how quickly today’s demands are, so if you have all of those five, you are going to watch the success of implementing synthetic data.
Matthew Heusser:
How fast should that run? Do you want to be able to create all the test data you test? Yes, down in an hour, a day,
Naresh Kumar Nunna:
Minutes. Actually, we’ve done a lot in Ally itself, the auto use case, which Pat talked about, you create a loan and then you age a loan, you make the payments, you miss a payment, it becomes a delinquent and there’s different delinquency cycles to that. For doing this, it’ll take organically. If you go through the application process, batch process, it could take days. Now, if you can simulate all of these events, you know what happens from a business perspective, it can be solved in minutes,
Matthew Heusser:
Right? I mean that’s one case, but to run through all of your test cases, to run through,
Naresh Kumar Nunna:
To run through all the test cases for all the permutation and combinations, we’ve seen the success. Let’s say if I take the whole regression cycle of running probably around a thousand to 2000 scenarios, usually regression cycles for this long is like a week or two weeks including the retesting defect, retesting defect, defect fixes we see in one to three days max.
Matthew Heusser:
That’s what I was expecting. That’s great. If I heard you correctly, you’re saying that you have your existing data, but we want to make sure we seed it with a diverse selection and we can use that to test for bias in our algorithms. Your example was a 250-year-old person taking out a loan. That’s the test for fraud. Did I get that right or is it to test for error?
Naresh Kumar Nunna:
It’s just for testing origination functionality. Where do you have a customer who is opening a loan who is 250 years old? If I’m talking about auto loan origination standpoint, we may not be existing.
Matthew Heusser:
I would think that would be fraud probably would be my guess.
Naresh Kumar Nunna:
It could be a fraud too. Yes,
Pat Rinaldo:
Definitely Matt. Maybe that’s what Naresh is, I think, trying to lean in on is there are anomalies that you see in the data on a regular basis, but you can’t always test for those. In terms of the boundary testing, in terms of edge cases, how do you solve for that and that is something that synthetic data gives us that even trying to manually create the data through the systems, it’s very difficult to hit all of those edge cases. Set aside the amount of time it would take the actual ability to do it, it’s not always there. I want to go back to something you hit on earlier because I think it really highlighted one of the major benefits of using synthetic data in your test environments. You talked about not only do you have to create it, but then you have to simulate how customers would make their payments, at what frequency, at what amounts, what does that look like over time?
We’re able to look at historical trends. We’re able to understand what that might look like on a regular basis and it stays within a pretty narrow range unless of course there’s something like a pandemic. If you think post covid in 2020, all of those things that we had looked at on a historical basis changed dramatically. We were at the forefront of leaning in and helping people to get through that time and so help programs that maybe had 1 or 2% of our customers previously suddenly had 20 to 30 to 35% of the customers in it, but at that point in time we didn’t necessarily have the ability to create a data set that now represented 30% of the customers taking advantage of help programs, but with synthetic data, we would’ve been able to simulate that. We would’ve been able to understand it ahead of time as opposed to having to react in real time and adapt on the fly. Now we have the ability to simulate through synthetic data any of these scenarios that might be well outside of what you would expect to see on a regular basis. Certainly 2025 is not disappointing us in variability as well, so it’s going to be beneficial in trying to stimulate some of the things that we might see over the next six to nine months.
Matthew Heusser:
That’s a great segue into what your vision is for what your company is trying to do with synthetic data and how do you know that you’ve succeeded?
Pat Rinaldo:
We’ve put a lot of investment already into strengthening our overall tech foundation, whether it be security, privacy controls, our data warehouse, and we’ve also put it into our engineering practices. We need to be nimble, we need to move quickly. The world changes faster than you can blink and technology needs to be able to roll with it and adapt. The last mile in my view in terms of really being able to be innovative from a technology perspective is being able to have the test data that you need so that everything else can move at the speed that it needs to move. Core for me is being able to allow our engineers and our agile teams to move unencumbered by test data constraints. That’s the first one, looking at our flow metrics to know that the throughput in our agile squads is going up, looking at the turn time on initiatives to make sure that that’s improving.
That’s all around the speed. Then you have the efficiency ratios, how much testing is required for how much engineering, how much of the team’s time is spent actively working versus waiting for test data creation or other things, so anything around efficiency and then lastly, what’s the escape velocity for defects? How much is making it into production? I expect to see that go way down because a lot of times when we do retrospectives on that escape velocity, what made it through the testing phases, it’s often the edge cases. It’s often the things that were outside of the test data set and when you summarize that speed to market efficiency in the use of our engineers and then quality increases because we have a full spread of test data across all of the things that we need to validate. Those are the metrics that we’re looking at to see the impact of using synthetic data
Matthew Heusser:
Makes a ton of sense to me. I mean I was impressed. One of the things Michael and I like to talk about is reducing that final inspection regression test process because as soon as your regression test process takes too long, then everybody else is waiting for feedback and they’re writing new code that’s untested. That alone should get you the results, but if you can improve the quality of the testing at the same time. Yeah, so how’s that shown up Pat? I mean you’ve been doing it for a couple of years now.
Pat Rinaldo:
We’ve been working with Qualitest for a couple of years. I would say we’re just now scaling out our synthetic data into the application space. We’ve been in the reporting analytics space for quite some time with it, using our own models. That’s been extremely successful to be able to get full coverage. If you think about a lot of the analytical tools and the reports, they’re designed to handle all the cases that you might see over multiple years, but those don’t generally exist all at the same time. We’ve been very successful in being able to generate the data required for that testing and we’ve seen improvements in all the metrics. We’ve seen significant reductions in defects that make it through on the analytics side and a lot of speed in terms of how our teams are able to develop. In the application space, the operational space, we’re expecting to see the same results. It’s a bigger hill to climb because you have to simulate, Michael was talking about it earlier, all of this data, it’s not just landing into a well-formed data warehouse. It has to go back into these operational systems and that’s a bit of a bigger hill to climb still early in the journey for us in terms of are we going to see the results across those three dimensions that I thought, but all early signs point to, yes, we’re going to see it.
Matthew Heusser:
That brings us to today. This topic, a little bit like AI and ML, seems to be changing on the daily. If you were to look into your crystal ball, Naresh, what’s next? What’s the future outlook for synthetic data?
Naresh Kumar Nunna:
I think it’s more of a use cases, where and how you use it. We’ve already seen synthetic data in application development testing and we’ve seen success there. I think there is more effort need to go into how do I train my models to be more predictive? That’s definitely one area where we see a lot of room to grow and most importantly go beyond structured data. Today’s data is not structured data anymore, so we have data coming from social networks, IoT devices, smart devices, robotics, so it’s all going to be unstructured data, which is something not usable like we have been used to with the tables and columns and schemas. The other area where we don’t have enough material technology to do that today, but there’s a lot coming in to support this modern applications. Well, we’ve also seen creatively one of the customer that heavily regulated customer, they want to move to the cloud including the core applications.
They’ve done the peripheral applications. They also want to migrate the core applications as well. Now their concern is, okay, can I just migrate it? I’ve already done for these peripheral systems, can I just go and migrate the core systems as well? Business was not comfortable to do it because it’s heavily regulated. They have to go through all of these process again, they don’t know how easy it’s going to be, the integration standpoint, so the idea was, okay, you don’t have to put the real data first. Why don’t you put synthetic data, shake out the whole environment, get certified by audits, start with internal auditors, get it certified and if they feel comfortable, then you incrementally migrate these applications. These are the cases we have not seen this before Now synthetic data being used to test the new applications, new infrastructure, new platforms. Then build that confidence for the customers or business, so that’s also on the radar, which we have seen a significant uptick.
Lastly, the testing for these SaaS products and cart products, and these are always like black boxes, especially if you take COTS product, know very little about those products. They don’t necessarily expose their backend data models and structures to you. Everything is hidden inside the code. How can you make your data still intelligent enough to give me a copy, which exactly comes out of these black boxes? That’s another area where we see, again, potential with more and more applications becoming SaaS oriented and more and more vendor locked products that some of our customers are using. We can use the initial intelligence from the machine learning models to learn from the data, learn from the business specifications and create it. Those are the four areas I would say we see a lot of interest in customers
Matthew Heusser:
And I will say that I do agree that across all the customers that I’ve been working with over the past 10 years, the regulations, the governance structures to locking down of the data so you can’t use production data to test has just been increasingly difficult or it has to be mass or it has to be encrypted or it just seems like a breath of fresh air. Anything you want to add, Michael Larsen?
Michael Larsen:
Sure. I mean most of what I was going to be following up on Pat just basically summed up for me, but then I like to basically come out with is I like to end these shows with the idea of saying you get somebody who either doesn’t know about this, is skeptical about it or wants to understand more, what is your elevator pitch to them to say, Hey, in 30 seconds, this is why this matters to you and this is why you should care about it.
Naresh Kumar Nunna:
We are at a tipping point. Technology is changing so fast, we are living in a macro economy. I have to have things cost effective. I need to have things delivered today, not a week later. In some cases I’ve seen I want this to be delivered yesterday, so the speed aspect is coming in. Continuous growing regulations and privacy is also being a burden by the lot of businesses. How can I focus on actually creating the applications, focusing on the customers? Let’s worry about the privacy and compliance. Lastly, how can you make it easily accessible? I don’t want these solutions because they’re so complex. These are machine learning and advanced analytics. How easily we can make these accessible via self service approaches, self service channels, those are the key points we have to consider or keep in mind that will definitely get you to the North Star what you’re looking for.
Pat Rinaldo:
For Ally, as I said earlier, technology is at the center of everything we do. It’s the fuel that drives our growth. We view that innovation happens at the intersection of three things, differentiated customer experiences, driving business value and using contemporary technology. The speed at which that technology is changing today means that our engineers need to move faster than they ever have and the one thing holding them back is not having the test data that they need to be able to prove to others that their solutions work. Synthetic data gives us the opportunity to solve that and it will be the underpinnings of how we innovate going forward.
Michael Larsen:
Well, fantastic. As this is always the case, if people want to get to know more about either of you or how can they get in touch with you if they want to know more, this is your chance to give your plug. Where can people find you extra resources, articles that might be of interest, et cetera?
Pat Rinaldo:
For me, they can certainly hit me up through LinkedIn. That’s the best way to find me.
Naresh Kumar Nunna:
For me, I have two. One is LinkedIn. Second is qualitestgroup.com. I’m always available. I’m an enthusiast in the data, so hit me on the LinkedIn. I’m happy to take up any questions and have follow ups with you.
Michael Larsen:
Fantastic. Well, I want to say again, thank you so much for joining us and again, to all of our listeners, we thank you very much for joining us on the testing show and we look forward to coming back to you again with another episode very soon. Take care everybody.
Naresh Kumar Nunna:
Thanks.
Matthew Heusser:
Yeah, thanks Michael.
Pat Rinaldo:
Bye.